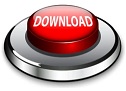
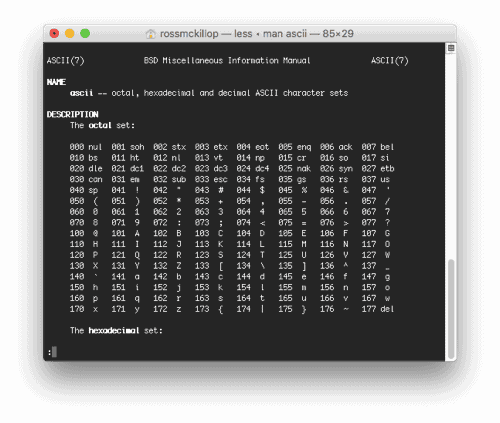
However, there is no suitable tetrahedral dataset for objective analysis and meaningful comparison of hexahedral mesh generators. Most of the algorithms tackling the hex meshing challenge use tetrahedral meshes as input or during some intermediate steps. However, there is no common dataset of input models, complicating the comparison of different methods. In addition, HexaLab collects the ouput hexahedral meshes of various state‐of‐the‐art methods. HexaLab is a visualization tool enabling the evaluation of hexahedral meshes by filtering elements and showing various quality metrics (corner scaled Jacobian, edge ratio, etc.). Recently, has introduced HexaLab to the meshing community. Three example models of HexMe ( ): The tetrahedral meshes faithfully represent feature points, curves (depicted in blue), and surfaces of the underlying CAD primitives.
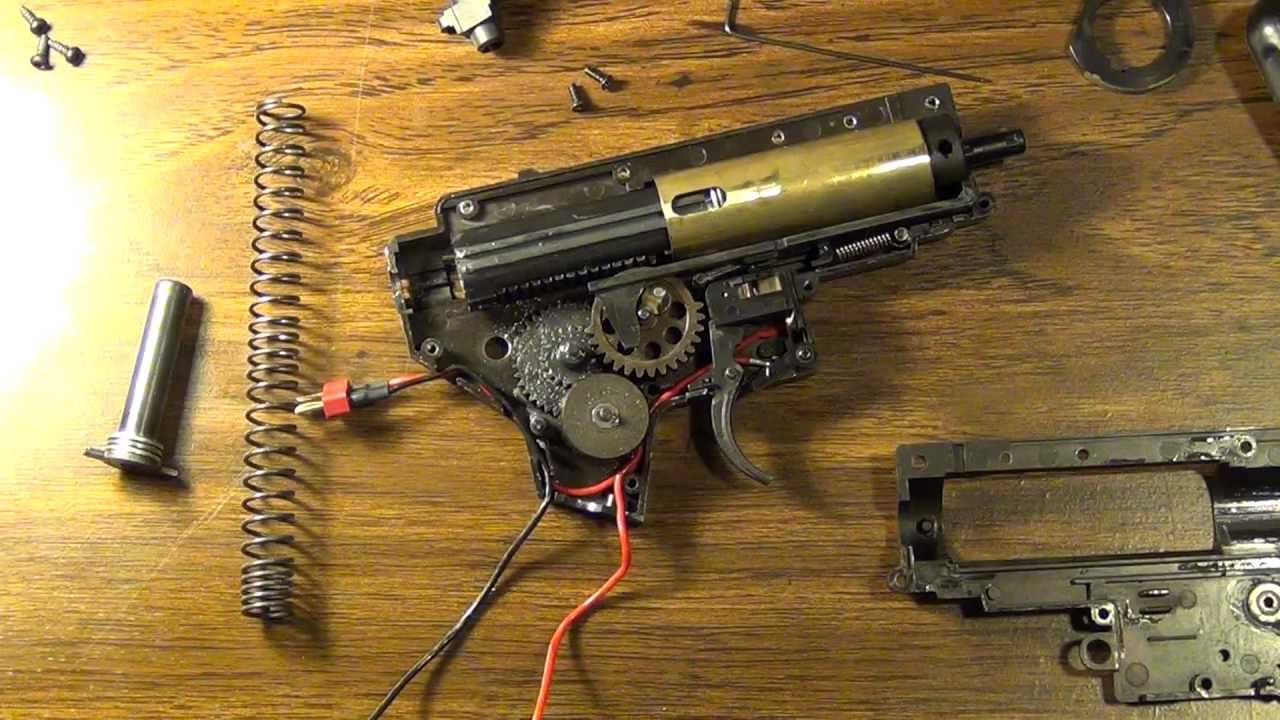
However, they are lacking robustness due to non‐meshable frame‐field topology, and other robustness issues in the generation of volumetric integer‐grid maps. Three‐dimensional octahedral frame‐based methods are a promising candidate to automatically generate high‐quality meshes.
#Gmsh verion 2 ascii full
Solely, octree‐based procedures can automatically and robustly supply a full hexahedral mesh, but with a quality far from ideal close to features (points/curves/surfaces). Automatic methods such as advancing front and polycube‐based approaches are neither guaranteed to be robust, nor assured to build high‐quality hexahedra. Industrial methods manage to provide high‐quality meshes, but they typically involve tedious and lengthy user interventions. In fact, there is still no automatic method robustly generating high‐quality hexahedral meshes for general shapes. Although numerous attemps have been conducted, there is still no satisfactory solution up to this day. In the last decades, the meshing community has been actively working on developing algorithms and tools, in order to implement a robust and automatic hexahedral mesher, c.f. Only for 19 of 189 tagged tetrahedral inputs all feature entities are meshed correctly, while the average success rates are 70.9% / 48.5% / 34.6% for feature points/curves/surfaces. We demonstrate the value of HexMe by exploring the robustness limitations of state‐of‐the‐art frame‐field‐based hexahedral meshing algorithm. A public GitHub repository hosts the HexMe workflow, where external contributions and future releases are possible and encouraged. The HexMe dataset is built with evolution in mind and prepared for future developments.
#Gmsh verion 2 ascii download
It is possible to download the whole dataset or select individual meshes by browsing the online catalog. The mesh generation pipeline is defined with the help of Snakemake, a modern workflow management system, which allows us to specify a fully automated, extensible, and sustainable workflow. For each CAD model, we provide three kinds of tetrahedral meshes (uniform, curvature‐adapted, and box‐embedded).
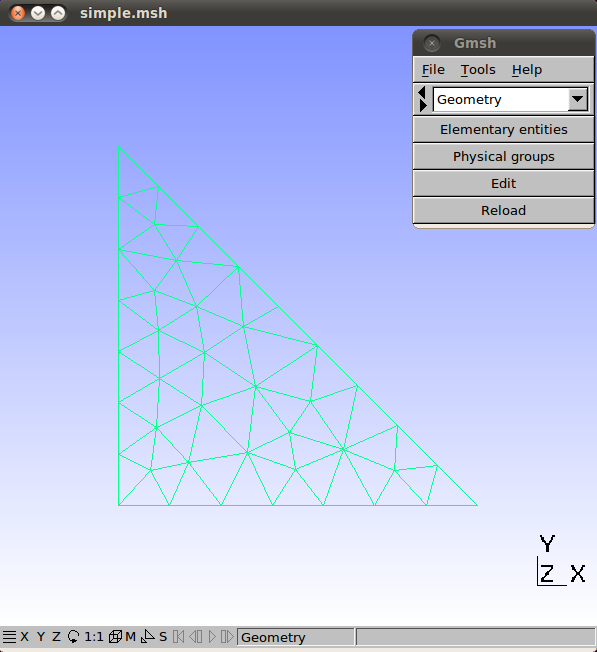
To highlight and label the diverse and challenging aspects of hexahedral mesh generation, the CAD models are classified into three categories: simple, nasty, and industrial. The tetrahedral meshes have been generated with Gmsh, starting from 63 computer‐aided design (CAD) models from various databases. The primary purpose of HexMe meshes is to enable consistent and practically meaningful evaluation of hexahedral meshing algorithms and related techniques, specifically regarding the correct meshing of specified feature points, curves, and surfaces. HexMe consists of 189 tetrahedral meshes with tagged features and a workflow to generate them.
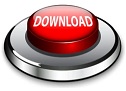